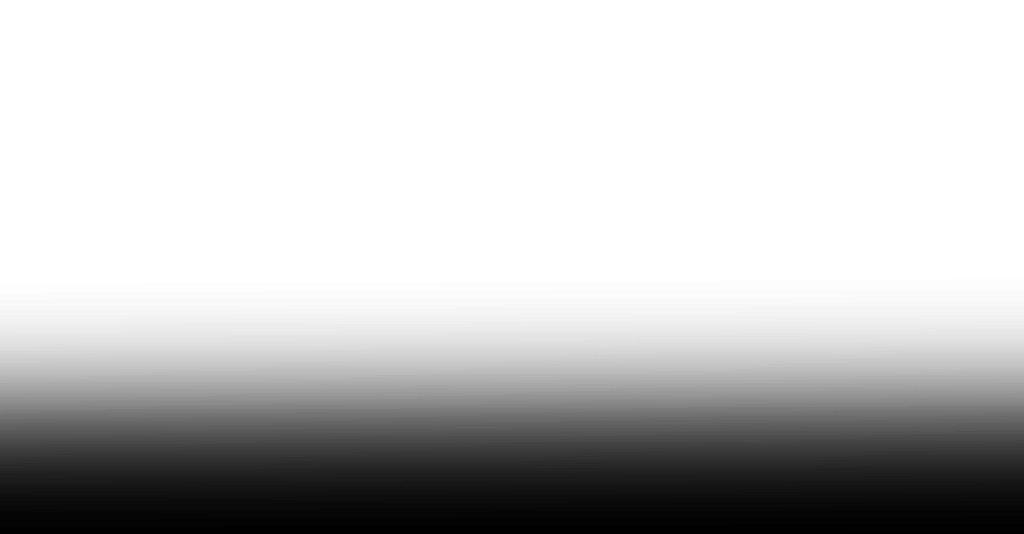
CASE STUDY
PROJECT BRIEF
Tracking interannual loss and accumulation of forest carbon is fundamental to conserving threatened tropical forests. In partnership with Microsoft Brazil, the Analytics Lab at CSP is leveraging machine learning models and massive volumes of remote sensing observations to track fine scale changes in forest carbon storage, advancing efforts to manage forest resources, forest biodiversity, and ecosystem intactness.
PROJECT DESCRIPTION
A primary tool for understanding and mitigating deforestation is the precise, repeated measurement of the mass of carbon stored in forest biomass. Forest biomass is an indicator of habitat intactness, health, and transformative disturbance. However, estimating forest biomass with manual, tree-level measurements at broad spatial or temporal scales is impractical and expensive. Thus, to track forest biomass at scale, we are applying the latest advances in cloud computing and machine learning utilizing a vast catalog of satellite observations, along with land cover and vegetation classifications. In partnership with Microsoft Brazil, the Analytics Lab at CSP is developing new automated workflows to generate maps of forest carbon stocks. Analyzing interannual variation in pixel-level carbon estimates provides opportunities for landowners and managers to plan for conservation, allocate resources for protection, and promote maintenance of intact forests that provide countless environmental services, including those beyond the realm of terrestrial carbon.